The Best AI Starts With Clean Data: Step-by-Step Guide
With a strong foundation of clean, well-governed data, you can make sure your data sets are as ready for AI as you are.
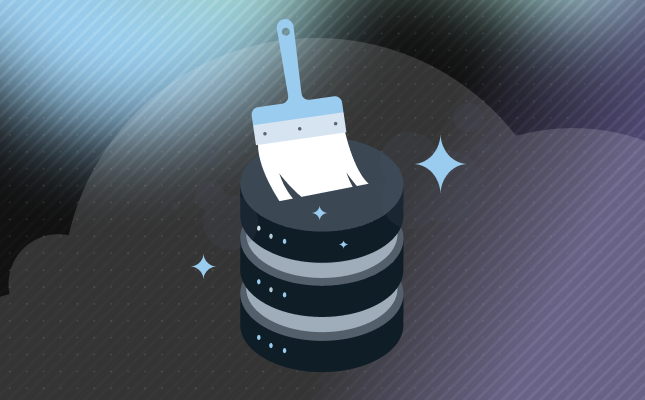
When it comes to AI, your data isn’t just an input—it’s the bedrock of every decision and insight your AI can provide. But what happens when the foundation isn’t solid? Just like building a house on shaky ground, trying to build AI on inconsistent or incomplete data can lead to unreliable results, misguided strategies, and an insecure structure.
Ensuring your data is clean and orderly is essential before launching any AI initiative. Part of this preparation is technical—and the AI Readiness Guide shared in our Community Forums covers best practices from Domo’s AI Labs team.
The other part is getting the right people, systems, and processes in place—and that’s what we explore below. By establishing a strong foundation, improving your data integrity and security, and fostering a data-quality culture, you can make sure your data is as ready for AI as you are.
Begin with better-quality data
Start with the right rows in your data set
Which data rows you need depends on how you plan to use the data—and starting with the right sample matters. At first, your data set may have some of the right rows, some of the wrong ones, and some missing entirely.
Sit down with the stakeholders involved and think concretely about what you want from your AI project. For example, if your goal is predicting employee turnover, you’ll need to consider:
- Who qualifies as an employee?
- What kind of turnover are you considering?
- What time period are you looking at?
You may need to delete rows of data or add more rows to complete your data set. This upfront work takes time—but it’s less than having to go back and prepare your data all over again.
Clean your data set
Data cleansing is like preparing your kitchen before you start cooking. It’s essential for keeping your AI effective and efficient. Begin with removing duplicate entries to prevent the same information from skewing your analysis. Then move on to making your data formats consistent. For instance, all dates should be in YYYY-MM-DD format to avoid confusion and errors in time-based analyses.
Cross-reference your data set with reality
Let’s go back to the turnover example—do the hourly wages of each employee make sense given the population’s minimum wage? Are there surprising outliers? If so, don’t just get rid of these values—investigate them. In this case, check the numbers with your human resources director. Even tiny typos can throw off your analysis.
Apply validation rules to find errors
Once your data has been cleaned, apply validation rules to automatically highlight potential errors. For instance, a salary field showing a negative number should automatically trigger a review. Machine learning models can predict typical error patterns based on historical corrections and automate fixes for these issues.
Improve your data integrity
Deal with missing data
Missing data can be misleading; it might not seem like a big deal until your AI starts producing biased results. You don’t have a complete picture of your data when your data set has missing pieces. Some algorithms can’t handle missing values, which means they’re learning from faulty information.
Develop a strategy that fits your AI’s needs, whether it’s using statistical imputation to fill in missing values or taking algorithmic approaches that adapt to gaps in data. Our data scientists walk you through their process in part 1 of our AI Insights livestream series.
Audit your data regularly
Follow up with regular data audits. Think of audits as detective work for your data, where you hunt down inaccuracies or missing bits that could chip away at your AI’s foundation. As mentioned, automated tools can help you spot anomalies, making sure your data stays pristine.
Establish data governance policies
Now that you have great data, you need to ensure its security. As you implement AI, set up a comprehensive data governance framework that defines who can access which data sets and under what conditions. This should include not only permissions but also tracking who accessed what data and when, to keep your organization accountable and compliant with data protection regulations.
Educate your team on data security best practices
Provide ongoing education and workshops for all employees about why data quality matters and their roles in maintaining it. You could also establish key performance indicators (KPIs) related to data quality and integrate them into performance evaluations.
Foster a data-quality culture
Engage your team
Involve your team in maintaining data quality. Encourage them to identify potential areas of improvement and suggest solutions. This not only improves your data but also helps cultivate a culture of quality across your organization. Celebrating these contributions can boost morale and encourage a proactive approach to data management.
Review and update your data practices regularly
Data requirements and technologies evolve, so your approach to data management should, too. Regularly review your data practices and stay updated on solutions for improving your data’s quality and security.
Listen—don’t ignore feedback from your people
Opening up a dialogue about data quality within your organization can lead to new insights and improvements. Encourage feedback and use it as a stepping stone to better practices.
Don’t stop here—keep learning about AI, your data, and Domo
Creating a solid foundation ensures your AI system—and the data feeding into it—is safe, secure, stable, and accurate. The steps outlined here will help you start strong, but consistently improving and adapting to new challenges and technologies are key to maintaining high-quality data—and getting better results from your AI.
Ready to dive deeper into AI data strategies?
Don’t miss our next webinar, “Implementing AI Safely and Effectively,” where we’ll explore advanced techniques for ensuring your AI initiatives are built on a foundation of quality and integrity. Sign up for the next episode of our AI Insights Livestream series.
Want more on data cleanliness?
Domo’s AI Labs team hosted the first part of this livestream series in May 2024. Watch the recording here—our data scientists will show you how to clean your data step by step.
What's Your Reaction?
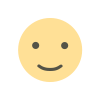
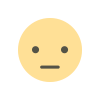
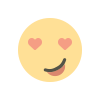
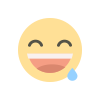
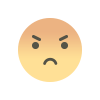
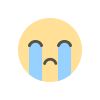
